Kubernetes migration services

Well-known K8s experts expanded to the Web3 world

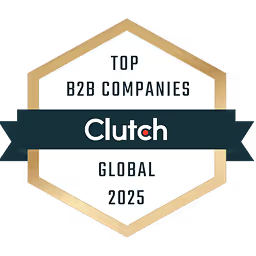
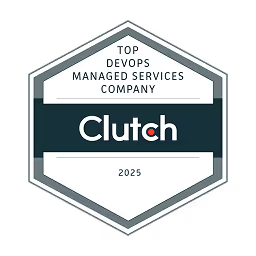
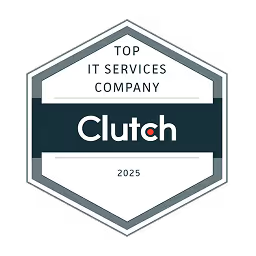
.avif)
.avif)
.avif)
.avif)
.avif)
.avif)
.avif)
.avif)
.avif)
.avif)
.avif)
.avif)
.avif)
.avif)
.avif)
.avif)
.avif)
.avif)
.avif)
.avif)
.avif)
.avif)
.avif)
.avif)
How do I migrate to Kubernetes?
The best way to conduct Kubernetes migration is to delegate it to the experts like Dysnix, who go through this process thousands of times. With our experience, you have fewer chances to deal with avoidable mistakes and prevent any other errors. We’ll be pleased to complete migration of any complexity and get your project on k8s rails with ease and no harm to your traffic.
What is a cloud migration service provider?
Alongside companies that are specialized in k8s migration, there are cloud migration service providers that make on-premise products and services cloud-based. The procedure of this process is quite the same as in Kubernetes migration, because you always go through the change of infrastructure paradigm from in-house servers to cloud-type servers. Dysnix, as one of the top cloud migration service providers, can help you with migration to the cloud using not just the Kubernetes migration tool. Please consult with us regarding your project to find the optimal solution.
How do I migrate from one cluster to another in Kubernetes?
Kubernetes cluster migration is more accessible than moving the whole infrastructure from on-premise to the cloud. But it has some complexities, too, such as moving without affecting the traffic. If you set up this condition, there are not a lot of ways to solve this task. For example, you can use the cloud DNS and internal load balancers to create a smoother process. For more information, check our blog and ask our specialists.